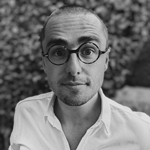
Our 27th workshop features a conversation with Christopher Barrie on “Measuring Media Freedom with Word Embeddings” on October 21, 2024, from 9:30AM – 11:00AM PT.
The Hoover Institution Workshop on Using Text as Data in Policy Analysis showcases applications of natural language processing, structured human readings, and machine learning methods to analyze text as data for examining policy issues in economics, history, national security, political science, and other fields.
>> Erin Baggott Carter: Welcome all, and good morning to the Hoover Institution workshop and using text as data in policy analysis. I'm Erin Baggott Carter and my co organizer Steven Davis and I are delighted to welcome you this morning to a presentation by Christopher Barrie of NYU, an assistant professor in sociology, who is going to speak this morning about measuring media freedom with word embeddings.
Just for a quick reminder about our format, please put any questions you may have in the chat box, and we'll call on you to ask them in person if possible, and if not, we'll ask them ourselves. The formal presentation will go until 10:30, at which time we are welcome to stay for an informal Q and A with the author, Chris, please take it away.
>> Christopher Barrie: Thanks a lot Erin, it's a pleasure to be here, and thank you again for the invite. Okay, so this is a paper that I've written alongside three co-authors, Neil Ketchley at the University of Oxford, Alexandra Siegel, whose work I'm sure many of you are familiar with, who works in similar domain to what we're focusing on in this paper today.
She's at UC Boulder and Mossaab Bagdouri, who's actually principal data scientist or one of, I'm not sure if he is the, or one of the principal data scientists at Walmart. His participation will become clear later on, a slightly odd affiliation granted for a paper such as this. Okay, so I'm going to show you, to begin with, a few front covers of Egyptian newspapers.
This is the front cover of Al-Ahram, this is the Egyptian newspaper of record, really. And it's from June of 2008, where they're reporting on a worldwide opinion poll that puts Hosni Mubarak, then dictator of Egypt for well more than about two decades by that point. Puts his opinion poll ratings or his trust ratings, 94%, saying as making him, as this headline puts it one of the most trusted leaders in the world.
Now fast forward just a couple of years to January of 2011, we see protesters in the wake of nearby revolution in Tunisia, begin tearing down posters of the very same Hosni Mubarak in squares across Egypt, starting from Cairo. Another two and a half weeks later, the protesters swelled to the many millions, with protesters now explicitly calling for the ouster of Egypt's, then so called president for life.
Now, what we see in the wake of these events is really interesting, we see this flourishing of somewhat, indeed independent news media in a way that was a stark contrast to everything that came before these events. Indeed, this is a front cover of one such newspaper that grew up in the wake of revolution in Egypt, which is criticizing then interim leader military general Tantawi for sounding too much like former dictator Hosni Mubarak in saying that he wouldn't let the actions of protesters jeopardize national stability.
Now, fast forward just another two years later in the wake of a military coup led by this man, Abdel Fattah el-Sisi. We see a kind of elliptical return to the status quo ante with 20 page investigations or supplements, in this case, in the same newspaper of record Al-Ahram from June of 2016, extolling the virtues of now President Abdel Fattah el-Sisi.
So why am I telling you all of this? Well, I'm telling you this because I think media criticism of governments, that is the way in which news media report on those in power, is really, really important to understanding several key phenomena within the social sciences. It's key to understanding collective resistance and its dynamics, it's key to understanding authoritarian regime behavior.
And more broadly, it's a key component of many democracy indices worldwide. But what I wanna communicate to you today is that this, I think, really important component, all of these things, something that's really important to measure, actually does lack really reliable forms of measurement. So what I'm gonna do in this talk is kind of get into the weeds of the technique that we're proposing in this paper for measuring media criticism.
And this is a technique that I think represents a real advance because, firstly, it's based on the text of newswires alone, we don't need anything more than that. We think overcomes several of the shortcomings of current approaches to measuring media criticism, several of which I'm going to provide an overview of in just a moment.
What's more, it can be extended to multiple different domains. That is to say that the basic underlying analytical technique here, that uses a form of word embeddings based analysis can be extended to multiple different domains outside of the measurement of media criticism alone. Okay, so I'm gonna go through in the first part of this talk how we've studied news media under authoritarian systems more generally.
I`m then gonna go through quickly some of those general approaches we have now to measuring media criticism. I'm gonna introduce what our case studies and our data are in this paper, and then I'm really gonna drill down on the method that we use and we're proposing in this paper.
As well as its particular beneficial components in comparison to what's come before it, and then go through some of the findings and validation steps that we have undertaken. Okay, so how have we studied news in democracies or in authoritarian systems? Well, the first thing to say is that in these kinds of political contexts, right, news media reporting on the actions, policy decisions of governments is not just reporting.
An authoritarian context, to be critical to having news media, or institutions of news media that are critical of the government can be understood as a type of protest. And indeed, there have been some really important contributions in sociology that conceptualize media criticism, is just that. And the question then is how can people mobilize collectively in order to resist in this way?
We also know from the political science literature, that there are strategic benefits to critical news media. Here the prevailing question is, if authoritarian governments have the capacity to repress critical news reporting, why is it that some is still permitted? And here the answer, is that there are actually strategic benefits to critical news media, because it performs an informational function that these governments otherwise wouldn't have in the absence of some kind of media freedoms.
This is actually what we see a lot today for contemporary authoritarian systems, if you like. Well, the third thing to say is whether we're looking at the political science literature or the sociology literature, indeed, the economic literature. A key component of what makes media criticism salient within the political sphere is when that news reporting is critical of the political executive, that is to say, the person or persons in charge of a national polity.
And that provides a key metric of the intensity of regime criticism that's going to be important when we come to. Some of the more when we come to the method that we use in this paper. But as I said at the top of this presentation, we're not actually very good at measuring.
What we argue in this paper is a really important component not only of democracy indices, but of understanding things like collective resistance of the functioning of authoritarian regimes and so on. So what do we do at the moment? Well, there are three main ways we argue in this paper that people have done this today.
First are these really, really rich qualitative accounts of newsrooms, and particularly newsrooms during EPSA, during periods of political transition. When journalists are kind of re understanding their role in the context of the renewed freedoms permitted them within these transitional context. But this obviously doesn't give us is any kind of quantitative metric of overall generalized changes, if you like, in the way in which news media are reporting on politics, on the actions of governments.
We also have these text based accounts of relatively small samples. So here scholars might take a sample of 500 or 1000 news articles over a ten year period, randomly chosen, using some kind of randomization technique. And then they will hand code these news articles for the intensity of criticism directed towards national or local governments, let's say.
And that provides us with some sense of overall change, though it's not very scalable, it's very labor intensive, right? And the third, and there's really a whole industry around these, within political science in particular, are expert surveys. Now, many of you will be familiar with these, these are things like the Economist Intelligence Units Index, like freedom houses, freedom of the World reports.
As well as the V-Dem's reports on democracy or the health of democracy worldwide. Now what's interesting about these modes of collecting data is actually they've come under some rather intense scrutiny in recent years. And part of the reason for that is there've been real question marks over the subjective dimension of many of the components of these measurements.
So for those of you who don't know, here's an example of how the Wielder Institute's expert survey scores are generated for the media criticism item. Which is a key component of their democracy, their overall democracy indices that they publish yearly. And so what they will do is they will ask individual experts, anybody in this room, I'm sure, and I've done these once or twice myself.
And they will ask them here, of the major print and broadcast outlets, how many routinely criticized the government. They were asked that question not just about the present day and about countries in general, but about a particular country for a particular year. So you might be asked in 2024 how free the news media were on a scale of 0 to 3, how free the news media were in Iraq in 1954.
And you would have to give an answer to that. Now, what's important to stress is that these are really the best way we have going of measuring this component of media freedom. So the point of this talk is not to direct or target any particular criticism at VDEM.
It's to emphasize that the current ways in which we tend to measure these kinds of items are arguably kind of lacking. So our approach instead overcomes what we deem to be three shortcomings in the current approaches to measuring media criticism. Which are, firstly, that they're extremely labor intensive.
If we're an individual scholar hand coding several thousand news articles, that's labor intensive. If we're organizing expert surveys across multiple countries, across multiple years, that's extremely labor intensive. Secondly, whether you're hand coding it individually or whether you're using an expert survey, these tend to be highly aggregated to at least the year level.
We get nothing more granular than that. And thirdly, as I've just argued that these are vulnerable to various forms of subjectivity bias by individual expert coders. Okay, so the question we're kind of asking in this paper is this, can we instead measure media criticism just using the raw text of newswires alone?
And what we argue in this paper is that, yes, we can, you won't be surprised to hear, and I'm gonna go through now some of the ways or the way in which we set about doing this. So what we do is we choose two, we refer to in the paper as change cases.
These are cases or countries that have witnessed significant periods of political upheaval and subsequent transition. So these are Egypt and Tunisia in the Middle East, North Africa, which is our focus, obviously, in this paper, in good part because that's where the data come from. But also in good part cuz this is actually somewhere where we've seen significant change in this domain, that is in the news media domain and associated freedom.
So what do we see in both of these cases? Well, we see in both of them political openings following the Arab Spring uprisings of 2010 to 2011. What we also see in both is a period of media liberalization that began from this moment onwards. And in Tunisia, at least until 2020, which is the end of our observation period, we see a sustained wave of democratization that follows these episodes of popular upheaval.
Whereas in Egypt, we see a reversion after the military led coup of the summer of 2013. Our stability cases may surprise some of you who are area specialists because in Saudi Arabia, for example, we did see significant elite level descent. Just as we saw significant popular descent in both Algeria and Morocco over various periods.
But we didn't see in any of these countries were any change in the actual regime type or indeed any really salient change, consequential change in media freedoms within these contexts. So we refer to these as our stability cases, okay? And here's what the V-Dem scores look like for these countries.
So this kind of matches with or aligns with what I've just described about these cases, okay? So in Egypt we see this large uptick in media criticism following the Arab uprisings or following the Egyptian revolution of 2011. Followed by a decrease to the status quo anti or indeed even worse in the aftermath of the coup of 2013.
Whereas in Tunisia we see this uptick in media criticism or the ability of news media to routinely criticize, as the question goes. Government, whereas in Algeria, Morocco and Saudi Arabia, we see relatively no change over the observation period. So this seems to align, right? With what we know to be the case about these countries.
Okay, so how do we go about trying to derive some kind of measure that is cheaper, less aggregated and less subject to vulnerable, to subjectivity bias? Well, we take this really fantastic dataset. Now, this dataset as you may have guessed by this point collected by one of the co-authors in this paper Moss Baghdadi, right?
It was actually started as his master's computer science project, where he tried to make a news aggregator for his home country of Morocco, and then got carried away, and made one for Algeria, and then one for Egypt, and then one for Saudi, and then one for Tunisia as well.
That, well, he's still doing this, by the way. And all in all, there's many tens of millions of news articles across hundreds of different newspapers, that he's managed to aggregate across these different news aggregation platforms for the Middle East and North Africa. So this is what they look like, many, many gigabytes of raw text data to which we as a team negotiated access with Mosab.
And we use these as the underlying data to validate or test, if you like, the method that we're proposing in this paper. And that's what I'm gonna really now drill down on for the second part.
>> Erin Baggott Carter: Before we get to method, this is all fascinating, thank you. Can you tell us a little bit more about how you think about potential bias about inclusion and censorship in these news aggregator websites, which are often surveilled by autopilot governments?
>> Christopher Barrie: Yeah, that's very good question. So they are surveilled by governments. We had this conversation with Mosab, and there were two or three times in which we're told that he received requests to take down information from the aggregation platforms as a result of copyright claims rather than any kind of broader political intervention.
The other thing to say is that all this is doing is crawling news from actual news websites within the region, all right? So anything that goes on there will, or most of it anyway, will end up on this aggregation platform. And I don't know of any additional interference that has taken place from governments that would bias things in one way or other.
But, yeah, I take the point. Does that answer your question there? Yeah, okay, so to get onto method, what do we do? We will use something in this paper with which I'm sure some in this room at least, will be familiar, and these are called word embeddings, but it's a particular flavor of word embeddings that we're using in this paper.
The basic underlying technique is a relatively old one now, at least for this fast moving domain, which is text analysis, which is to generate a glove based word embedding, an algorithm that was developed in 2013 by Pennington and colleagues. How does this work? Well, it's actually relatively straightforward.
So let's just imagine all of our text in our news articles. We take the unique vocabulary in that text, and that means every unique word that has appeared across the entirety of that corpus, all right? Then we assign each word an integer, just based on the order of its appearance, and then we essentially generate this matrix, right?
This matrix, Xij, which is the number of times word wi, let's say the word president co-occurs with the word wj. Let's say the word government within a given context window. And the context window here refers to the number of words within which we're looking for a co-occurrence. So normally this is as a rule of thumb, six or twelve words, because this provides us with the most signal for understanding kind of semantic meaning of words, that can be derived from their placement within a sentence.
So it would look something like this, right? In the end, we're gonna count up the number of times that the word executive in Arabic appears within a six word window of, let's say, the word minister, this is all made up data, by the way. And we're gonna build from this basic underlying foundation stone, which is a co-occurrence matrix, very simple way of converting text in some kind of numeric form.
Well, our goal is to do this, to weight each individual word such that if we were to project them onto some two dimensional space, that words that have a close semantic association would appear closer to each other than words that have less of a semantic association. So here we see the words ministers and minister appear close to each other, whereas the word tree appears further away.
This is what we would expect and what we would aim to try to calculate. And the way in which we calculate this is by using a GloVe algorithm. I'm not gonna get too deep into the underlying operations here, because what I wanna focus on is this alternative flavor of word embeddings that we use in this paper in order to generate our measures of media criticism.
So what do we do? Well, we generate what we refer to in the paper as a reference embedding. And all of this is a one time GloVe embedding of all of the text, across all of our countries, across all of our newspapers, right? And so we generate this, and we do this for vocabulary of size 30,000, so we choose 30,000 unique words to generate our embedding layer.
The problem with this approach is that if you could think it through, it's then very hard to make overtime comparisons, why? Because if you wanted to look at changes in the position of individual words, the weights of individual words, how close they are to each other overtime, you would have to repeat this process for every single one of your time units.
And actually training an embedding layer at least on your local machine, is quite computationally expensive. It might take from 2 hours to 24 hours if you wanted to do it. The second thing to say is that actually it's not identified, because if you think about it, you're estimating your embedding on a different underlying corpus of for each time unit.
So you can't necessarily make comparisons between the two. So here's where this new approach comes in. There's a really nice piece by Pedro Rodriguez and colleagues in 2023 in the APSR that uses this technique and introduces it as a way in which we can effectively use, excuse me, regression based methods within a word embedding context.
And the way in which it works is strikingly simple and really, really smart I think. What it does is it says, okay, if we want to make overtime comparisons, what we're gonna do instead of retraining an embedding layer at each step, is we're gonna induce a time specific embedding for our target word.
By simply taking the average of the words that appear around it from that one time reference embedding layer that we've just estimated, we only have to estimate once. So, here's an example of how that would look. So, here's a six-word context window. Some article is saying that someone stated that the name of the president here, and that's what the target that we're using throughout these analyses was in trouble.
Okay, so, we're not gonna get that much signal from the word stated that the was in, right? But trouble, okay, the president maybe is in trouble. We then average those, and we do that within each time unit for every single mention of that president, for that country, across all of the newspapers that we have in our corpus for that country, okay?
And that provides us with our time specific embedding of how the president, the figure of the president was being talked about at that particular moment in time. Okay, yeah.
>> Erin Baggott Carter: Just another quick clarification question. I noticed that you're not stemming these words in the slides here or reducing them to their root forms.
And I'm curious cuz when I worked the Arabic text a couple years ago, stemming in reduction to the roots was really just rudimentary at the time. So I'm curious, given how many word endings there are in Arabic, if that introduces level of noise in the analysis or how you think about that problem in the area in particular.
>> Christopher Barrie: Yeah, that's a great question. So, what we did in this paper, if I recall correctly, is that we only got rid of stop words because there was no additional, I'm going to say two things. There's no additional power given by stemming. In fact, that kind of dilutes the semantic meaning of a lot of the text, because then you're not necessarily looking at the word in the direct context in which it's being used, which might be in, for example, in the passive form or otherwise.
And that would therefore go undetected if you were to stem things. And so, you want to keep as much of that signal in the text, if you possibly can. And that's why we didn't stem in this case. I don't know if you've had different experiences, but that was the approach we took.
The thing that you do need to think about in this case is, is the transformation that happens at the end of the analytical pipeline. So here, to reiterate, we're taking mentions of our president. We're taking the words around that president word. We're averaging them within that time unit.
And then, this transformation matrix at the end is effectively down-weighting words that don't give us a huge amount of signal, right? So, we're taking out stop words and staff, at least, at least some of them. But in this example, which is a toy example, if we take the sentence stated that the president was in trouble, and then we're averaging those, right?
Well, transformation matrix is effectively upweighting the trouble vector because it's a less common word and it's probably giving us a little bit more signal, okay.? The final step in this whole thing, in this whole pipeline, is that we need something to compare this to. So we have this vector, right, of how an individual present is being talked about within a particular time period, but we don't necessarily have anything to which we might compare it.
And what we wanted to do in this paper was to provide a kind of very tractable, generalizable technique for measuring that kind of thing. We chose the most simple technique we could think of, which is essentially go back to the reference embedding, take the word for opposition and subtract it from the word for support.
And then, our similarity metric between our individual year-week leader embedding for an individual president is simply the cosine similarity between that and this criticism index. What we're referring to in the paper is our criticism index. If it comes, if our year week leader embedding is closer to the support end of this criticism index, we understand this individual president to have been spoken about in more positive terms or less critical terms, and vice versa.
And that provides us, in the end, this whole long pipeline with our weekly embedding or weekly metric or measurement of the criticism directed at an individual president within a given country over time. Okay, I have several results and then validation steps that I can walk you through. But in the interest of time, I think I'm going to focus just on the main results, and then I'm going to go through, I can go through some of the further validation in what comes after and in the Q and A.
Okay, so what do we see? Well, to remind you, this is what the VDAM metrics of media criticism look like for this time period. And what we get in the paper is something that looks not dissimilar. Now, we see more movement around the lines than we might have expected for our stability cases.
But we see in Egypt and Tunisia are two overtime estimates that seem to track relatively closely the widem estimates of these metrics. So in Egypt, we see this uptick in criticism after 2011, followed by a decline whereas in Tunisia, we see the sustained uptick in media criticism that is sustained throughout our observation period.
I realize now that I've reached time. So, I think I'm gonna stop here before going through any of the further steps that we took and listen to your questions. Thank you very much for listening.
>> Erin Baggott Carter: Fantastic, well, thank you, Chris. This is a really fascinating presentation. And audience members, please put your questions, any that arise in the q and a box, and we'll be happy to call on you or to.
Yeah, Steve.
>> Steven Davis: So, I wanna make some conceptual observations to start with. First, I want to draw the distinction between the volume of media criticism, which I think is what you're measuring, and the ability and willingness of the media to criticize the executive. Those are related, but they're not the same.
And they're not the same for a few reasons. One is that there may be less grounds for criticism of some authoritarian executives than others. So, one example that comes to mind is Lee Kuan Yew, in the early decades of Singapore, widely regarded as an authoritarian leader with a, let's say, let's just say, a heavily regulated press.
But it's also the case that his party did a remarkable job of fostering relatively strife free improvements, and he. Economic prosperity. So there was less reason to criticize. And if you were to take your type of measures and apply them to singaporean cases, I suspect you'd find little criticism.
But it's probably both because there was repression and because there was a relatively successful autocrat. That's kind of .1 about why these concepts differ. And if I think, then I wanna turn to the US context. So there's lots of indication that in World War Two, the media went lightly on criticism of government policy, government performance, and so on.
And as I understand it, it was because it was viewed as part of your patriotic duty at the time, given the nature of the war in which the United States and its allies were involved in. So that might have been, there was some overt censorship, especially of military related activities, but much of it was, I think, undertaken willingly and voluntarily, and viewed as a positive thing by members of the media.
You can think about the media's unwillingness to lay bare John F. Kennedy's affairs and how it might have compromised the performance of his function during his presidency. I think it's pretty clear that the legacy media in the United States had a very different willingness to criticize President Obama during his first term in office in particular, and then their willingness to criticize President Trump.
And so if I were to take your approach and apply it to those various instances, you'd certainly see changes in the volume of criticism. But it's not clear what that's telling you about the ability of the media to criticize the executive. And you could go on and on, I mean, we've recently seen in the United States really an astonishing willingness of much of the mainstream media to ignore President Biden's cognitive decline.
Now, that wasn't overt government censorship. There was no doubt some pressure applied. There was no doubt some norms that were enforced across members of the media or pressure. But that's different. So I think that's a fundamental distinction. In my view, what you're measuring is the volume of media criticism, which is an outcome of multiple things, including the level of repression of the media.
So I have a second, very different comment, but I think I'll let you respond to that one first.
>> Christopher Barrie: Yeah, it's a great point and very well taken. So I would say one main thing in response, that what we're proposing in this paper, we do talk about this in the discussion section.
What we're proposing in this paper is not some kind of magic bullet that can measure media criticism without any kind of noise or error. What we are saying is that combined with case knowledge, we can make a convincing case that this provides us with a more granular measure of media criticism than we otherwise had a less grand claim, but I think a more honest one.
So that's to say in the Egyptian case, and I have taken this, I've received similar comments from different audiences, some of whom have said, for example, well, the reason there was less media criticism of the Assisi in Egypt is because his government was performing better. So what you're capturing precisely as you were saying, Steven, is not mere criticism alone.
It's also about the performance of the government. What we need to do in those, or to kind of rebut those kinds of claims is combine what we're measuring here with some good amount of case knowledge. So what do we know about Egypt after 2013? We know that the economy was tanking.
We know the repression escalated. And if we were to compare that to contexts like Singapore, we wouldn't be able to make the same arguments. So if we were to see media criticism go down drastically over that period, I don't think we could reasonably make the same claim, of course.
So I do think that this needs to be used with some circumspection, some caution, and it does need to be combined with a healthy dose of common sense and knowledge about the cases that you're studying. But the point is well taken. I think we could do well to do better to make clear that we're not explicitly recreating a measure of what V mem is measuring.
I think you're absolutely right when you say that. Just to follow up on that.
>> Steven Davis: So just make it clear.
>> Christopher Barrie: Yeah.
>> Steven Davis: I'm not making a criticism of your measure of media criticism. I think it's very useful to have that volume. But the case study approach is useful, but you could be more systematic about it.
So I could suppose I implement your algorithm on dozens of countries around the world over decades, okay? And then I take standard measures and let's say economic performance measures, cuz those are the most widely available, comparable across countries, GDP growth, unemployment rates and so on. And then I construct an index.
And you might wanna limit this part of the analysis to countries with relatively free media, or you might not. But anyway, think about regressions of your kinds of measures on economic performance measures over a large sample of time periods in countries. And if you did that, for example, in countries that are widely acknowledged to have relatively free media, you would then get an index of the criticism level as a function of economic performance measures that would arise in a free media setting.
That's my shorthand here. And it would then become the gap between the volume of media as you measure it and the predicted value in a free media setting that would be a measure of the repression of criticism. So there is a way to kind of. It's another layer on top of your analysis, but there is a way, it seems to me, to get from your existing measure of media criticism to something that actually measures suppression of criticism at scale in an algorithmic way, which I take to be your ultimate goal.
And based on what you said at the outset of your discussion, when you describe the literature, it sounds to me like that would be completely new and a major innovation relative to what's already out there.
>> Christopher Barrie: Yeah, I agree with that. It's a very good point. So we've thought through some ways of doing this, one such way, effectively, what would be useful, right?
Is if we had some Egyptian news media outlet that had its office in London and was not subject to any kind of political intervention, right? And we had this before and after. We don't have that. What we do have our Tunisian news media outlets that also report on Egypt, right?
So we could use the Tunisian news media outlets in synthetic diff and diff context to try to get precisely at what you're referring to. I think, Steven, which is a kind of causal estimate of the effect of, given an episode of political repression in In this case, the coup of 2013 in Egypt on the extent to which news media are critical of the executive.
I can quickly whiz through all of these slides, and we get that estimate here. So here we're treating the Tunisian news media as our control, right? What would happen where Egypt would be had the coup not happened? And we can get this kind of causal estimate of this.
As you can imagine, this is kind of slightly experimental, still kinda noisy, but that's what we're working with.
>> Steven Davis: Yeah, so this is in the direction I'm suggesting, and this is useful, but I was suggesting something which is more global in its orientation and relies on averaging across countries.
And it would open up, I mean, it would open up the systematic exploration of things I think Aaron has done in other contexts, which is, when do authoritarian regimes decide to repress more and less? I mean, that's a strategic dynamic consideration as well.
>> Christopher Barrie: Yeah, yeah, point taken.
It's a really nice idea, something I'll think about for sure.
>> Steven Davis: And another much smaller comment, and you described in your review of the literature. One approach is to get a bunch of human beings who are presumably trained or somewhat expert, read several hundred or 1000 or so articles, and assess them subjectively as to the extent of criticism of the executive.
And as you rightly noted, that's a labor-intensive approach, although I've done a lot of that kinda work. It's not that labor-intensive with a small team of trained people. But the thing I wanted to ask you about is that approach is also eminently scalable. If you have a thousand, in my experience, a thousand trainings on newspaper articles of something, I presume that they're doing something like the extent or the tone of coverage of the executive.
So it basically boils down to a scalar value of criticism, either volume or sentiment or both per article. That's scalable using machine learning methods. And I'm not saying it's better or worse than the approach you've taken, but if there are already, I don't know how deep this literature is.
But if there are already multiple teams that have done that kind of thing with human readings, then you can build machine learning models and train them, test them out of sample and scale them up. That's a different approach to the very similar set of issues. But it has many of the advantages that you noted for your approach.
>> Christopher Barrie: Yeah, so I would agree, again, we tried to answer a question that was-
>> Steven Davis: Yes.
>> Christopher Barrie: So we tried. I would agree that it's scalable. I would disagree in that it does still require some resource. When we set out on this project, we only had resource to get the data.
So, what we did was we entered to hire a few people in Egypt to label some articles. So, we got them to label these articles. And we do see a trend using BERT that looks not unlike. So that's on the left-hand side, looks not unlike our end results.
We then used the same training data for Tunisia, and it was kind of less successful. So I do think you would have to have country-specific labels. You couldn't just train in one country and then kind of use it cross-country in that sense. We also tried GPT-4, which I disliked for many reasons, but I did it because I knew that reviewers were gonna ask.
>> Steven Davis: People would ask, I'm with you.
>> Christopher Barrie: Yeah, which kind of, like, works as well. But for all of the familiar reasons, if it's not interpretable, it's probably not going to be accessible in a year's time. It's kind of expensive. I don't think it's a way forward.
>> Steven Davis: All right, good.
You're already thinking along these lines, and so I'll stop there.
>> Christopher Barrie: Thanks great questions.
>> Erin Baggott Carter: Fantastic, just one question before we move to a few in the Q and A box. So I am curious. I think this is a really fantastic area, looking at how independent or quasi independent media cover dictatorships or cover constrained autocracies.
But my suggestion to you would be, I think you should look at variants, right? So in these news outlets, there are absolutely newspapers or media outlets more broadly, that are government-owned. And so it's definitely the case that many autocracies permit criticism of themselves, right? And they do so in a way that fluctuates over time, right?
So, you'll see a few coverage right before an election of the government. You'll see real clamp downs and sort of standardization of coverage in sensitive moments in the most oppressive autocracies. So, I think that what's interesting really would be the gap between media outlets that you believe are more independent or quasi-independent relative to that known autocratic baseline.
So I think it would be really interesting to separate out your corpus by the source of the media. And I think there's so much interesting stuff you can discover there, right? So one media outlet, first of all, when do they step out and actually start breaking ranks with the state, the state-owned or state-run publications?
When do they break out and sort of when do you see a coverage gap emerge in regime criticism? And what happens after that, right? So, after the first outlet steps out and begins to criticize or deviate from, like the government line that you can establish in your corpus if you just label which ones are state-owned, what happens after that, right?
Do other ones follow? Do you see these sort of broader changes in the ecology and media criticism? One really interesting example would be the fall of Ben Ali in Tunisia, right? So there we had a case study in that in our book, and it was kind of stunning, actually.
So, even the state-owned newspapers permitted tons of criticism, so honest propaganda. So lots of criticism of admitting regime failings right up until the day that he fled. But what was the independent media doing? Were they pushing that? Were they just thinking about that dynamic, sort of like this broader environment of different media actors?
And getting Molly Roberts has really fantastic work on bloggers in China. So what she finds is that after someone posts something that's really critical and they get taken down, they'll go and they'll do even more critical things, right? This sort of gets them angry and they want to do more sort of reporting and criticism.
So I'm just really curious thinking about that environment of these actors acting in concert in this broader environment. I'm curious to see if you've done more with that or how you think about that, yeah.
>> Christopher Barrie: Yeah, it's a great observation and question. So the short answer is we did, and we dumped it.
That's not to say that's an uninteresting question. I think precisely the opposite. Originally we had bisected the analysis by saying, okay, what are the newspapers that were state owned or formally state owned, and what are those that are kind of nominally private or independent right now? A confusing dynamic here is that those newspapers in Egypt, for example, that are private/independent.
You'll also find that there's somebody on the board who is the cousin of somebody who was in the executive before 2011. And things become a bit murkier once you look into what is actually going Going on behind the scenes, we had a similar intuition to your own Aryans in the sense that we thought it would be super interesting to look at first movers.
What we expected to see is that private newspapers were slower or independent newspapers were slower to begin criticizing or. Sorry, to kinda fall into line after an event like the coup summer 2013 in Egypt. And actually, what we saw is there was very little difference between the timing of the shift in tone of news media reporting in the aggregate, right?
As you say, we could also drill down at the outlet level into differences in reporting, and I completely agree that that would be super interesting. Difficulty here is data sparsity and a number of instances. That is to say, for a lot of the big newspapers, we have a full record over our observation period.
For a lot of the smaller outlets, it becomes a lot more patchy when the crawler has broken down to the news aggregation platform, to go back to your previous question. And that means that there are gaps in our data, which means that it becomes quite difficult without doing some kind of imputation procedure.
Which I'm a little bit hesitant about, to get reliable estimates at the outlet level. That's not to say it wouldn't be possible, it's just with the data that we have, it's difficult. So yeah, those are the two parts of my answer. I wish we could do it, but with the data that we have, it's tricky.
>> Erin Baggott Carter: Okay, thank you. Well, let's move to a question by Miguel Lopez in the chat box. Do the various sources of media carry different weights in calculating the score given readership, audience reach? Also, how are the social media sources coded, Facebook and TikTok, things like that?
>> Christopher Barrie: Yeah, that's a very good question.
Okay, we don't introduce individual outlet level weights. That is to say, we don't weight the signal that we're getting from the text alone by the readership of the audience? What we do is we do something that kind of resembles that. And I say it resembles that because effectively what we're doing is we're sampling a percentage of each individual outlet for each individual time unit.
And the big newspapers will have more contribution to that sample because they're bigger newspapers, right? By definition, they have more pages, they have more news articles. And as a result, they do contribute more signal, they contribute more of the underlying training data, if you like. As for social media, we ignore it, this is based on newswires alone, we don't get into social media.
That's not to say that you couldn't do something like this with social media. You would need some kind of reliable random sample of a given social media platform from a given country over a given time window. And that is something that's very difficult to get, as I'm sure you know.
But that's not to say that you couldn't extend this technique to social media if you had such a sample.
>> Steven Davis: I've got another comment of a different sort, it's on the scalability point, and also related to transparency and simplicity. It'd be really useful to know whether you can recover something that looks broadly similar to the outcome of your preferred method with a simple term keyword count.
And the reason that's potentially powerful is it's easy to implement that across dozens of countries over decades, that's kind of the whole. One of the key things about the policy and certainty enterprise that Nick Bloom and Scott Baker and I have developed is it's tremendously scalable across countries and over time you need the full text to evaluate its performance.
But that can be done at a modest level, either with human readings, which is the approach we took in your case, you could benchmark it against the outcomes of your algorithm. So it's an open question if I just went to something like ProQuest Factiva, whichever of these sources covers Arabic newspapers well.
Can I devise a simple keyword search approach? Some of these interfaces allow for Boolean operators and proximity searches, which would be extremely useful in your context. Because then you could get closer to something which is simple, but in the spirit of your complex undertaking. Where you're looking for criticism words or approbation words within proximity of the appearance of the executive, and then count the instances though.
Can you get something like this? If so, then you're really in business for scaling up.
>> Christopher Barrie: Yeah.
>> Steven Davis: It's partly a strategic consideration, now you can hear, like from Aaron's questions and from your own remarks, it's a lot. Let's compare this to various case studies. But if you could scale up your data by an order of magnitude, then we're in more in the business of generating and testing hypotheses on data at scale of a sort that simply isn't feasible with other methods.
So I would encourage you to give that a try. It's a different technology, but it's much simpler. It's much more scalable than what you're currently doing. In effect, in principle your approach is scalable, but in practice it requires access to the underlying text at scale. And that's simply either infeasible or cost prohibitive, whereas these keyword search methods are not, they can be implemented at scale.
I mean, there's probably do this for more than 40 countries now on our policy uncertainty website, some going back more than a century.
>> Christopher Barrie: Right, yeah, I hear you completely on that. Yeah, so where I completely agree is that, yes, you need the underlying raw text in order for this to be possible to an extent, right?
Well, I'm gonna say two things. Once you've estimated the word embedding layer here, reference word embedding layer, you're doing something not dissimilar from just counting words, right? You're just counting words around the target word. You're looking up their weights and you're averaging them. It's maybe like a step further along some analytical pipeline.
It's just as fast, basically, I mean, in seconds you can estimate what you're seeing on the screen right now once you have the reference embedding layer. The second thing I would say is that you kind of need the, oops, you see balloons on my screen? That has to do with your settings, but that's okay.
Yeah, okay, I need to look at it.
>> Steven Davis: We know a good point is coming now.
>> Christopher Barrie: Exactly, got excited. So the other thing to say is that you only need the underlying raw text in order to estimate the reference embedding layer. Now there are helpfully some Of these kinds of ALC embedding layers that have been provided to us.
So Elisa Wirsching, who was a NYU PhD, along with Arthur Spirling and Pedro Rodriguez and maybe I've missed a co-author, in political analysis, provides these for many tens of languages around the world. And you can use these as a kind of utility embedding layer for the given target language across national context.
Now, if you have these, then, of course, all you need are the context window words around your target word. And I don't know cuz I haven't used Factiva data, but maybe you can get something like that without getting access to the full underlying raw text. And what we did, just to go one step further with this answer, we essentially generated a bunch of synthetic articles using here GPT-3.5.
We prompted the model to generate just 500 critical news articles about this made up political figure, polit-fig, and 500 that are not critical in these languages. And we just implemented exactly the same technique that I've described to you. And what we see is something rather encouraging. Across all of these languages, it looks like our technique checks out.
That is to say, after time 0.0 this made up time point after 500 runs, we see our estimated criticism for each of these languages decrease in a meaningful way. So it does look like it is potentially scalable. As I say, it just needs that reference embedding layer. And after you have that, it's extremely quick.
And so-
>> Steven Davis: Yeah, so-
>> Christopher Barrie: Just a second part of my answer, why is this better than keyword searching or some kind of more familiar form of sentiment analysis. Well, I'd say it's better precisely because you're not relying on the appearance or otherwise of a set of words in a dictionary.
Because we can try and elaborate a dictionary that tries to capture criticism, but it's not gonna capture in the same way these kind of semantic associations, obviously, that we're seeing in text with- No, that's- The use of technology.
>> Steven Davis: I'm not so sure that's right. You have the design of the dictionary, so you wanna optimize.
The question is can you optimize this. And just to be clear, when I talk about dictionary here, I'm allowing for proximity searches, so it's more than just counting keywords.
>> Christopher Barrie: Okay, okay, okay.
>> Steven Davis: Because some of these platforms will allow you to search for other words within a proximity, some don't.
And the ones that do allow you to get very close, as you already pointed out, to what you're currently doing once you've got, I guess, your terminology for the embedding layer. So yeah, I would really encourage you to explore whether this is-
>> Christopher Barrie: Okay.
>> Steven Davis: A feasible route, because if it is, then there's tremendous scalability here of what you-
>> Christopher Barrie: Yeah, okay.
>> Steven Davis: And also great simplicity to the point where others can just go and run with. You can tell them, this works pretty well, doesn't perfectly replicate our preferred approach, but it gets 95% of the way. If you wanna implement it on your language, your country, your time period, there's reason to think that it'll give you something sensible.
I just-
>> Christopher Barrie: That's it right there.
>> Steven Davis: It's worth thinking about that and pursuing that angle.
>> Christopher Barrie: Yep, that's well taken. It's bulleted here as explore, underline, so I will do that, thank you for that.
>> Erin Baggott Carter: Fantastic, well, unfortunately, we are out of time for today. There is one more interesting question from Hoyt Bleakley in the Q&A box.
Hoyt, please do feel welcome to stay for the informal session, which begins right after this. Thank you, Chris, for a fascinating presentation on some cutting-edge methods and really interesting applications, so thank you for that. And everyone is welcome to stay after for the Q&A session. Thank you all for joining the Hoover Institution workshop on using text as data and policy analysis.
>> Christopher Barrie: Thanks so much, Erin, thank you, Steven, really great questions, thanks both.
ABOUT THE SPEAKERS
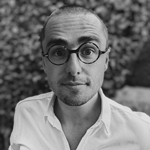
Christopher Barrie is an Assistant Professor in Sociology at New York University. I am a political sociologist of conflict, communication, and political attitudes. Methodologically, I specialize in natural language processing techniques, language models, and the repurposing of digital trace data for social science research.
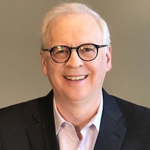
Steven J. Davis is the Thomas W. and Susan B. Ford Senior Fellow at the Hoover Institution and Senior Fellow at the Stanford Institute for Economic Policy Research. He studies business dynamics, labor markets, and public policy. He advises the U.S. Congressional Budget Office and the Federal Reserve Bank of Atlanta, co-organizes the Asian Monetary Policy Forum and is co-creator of the Economic Policy Uncertainty Indices, the Survey of Business Uncertainty, and the Survey of Working Arrangements and Attitudes. Davis hosts “Economics, Applied,” a podcast series sponsored by the Hoover Institution.
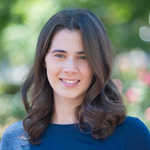
Erin Baggott Carter is a Hoover Fellow at the Hoover Institution at Stanford University. She is also an assistant professor in the Department of Political Science and International Relations at the University of Southern California, a faculty affiliate at the Center on Democracy, Development and the Rule of Law (CDDRL) at Stanford University’s Freeman Spogli Institute, and a nonresident scholar at the 21st Century China Center at UC San Diego. She has previously held fellowships at the CDDRL and Stanford’s Center for International Security and Cooperation. She received a PhD in political science from Harvard University.