Our 29th workshop features a conversation with Andrew Hall on “Investing in Political Expertise: The Remarkable Scale of Corporate Policy Teams" on December 9, 2024, from 9:00AM – 10:30AM PT.
The Hoover Institution Workshop on Using Text as Data in Policy Analysis showcases applications of natural language processing, structured human readings, and machine learning methods to analyze text as data for examining policy issues in economics, history, national security, political science, and other fields.
WATCH THE WEBINAR
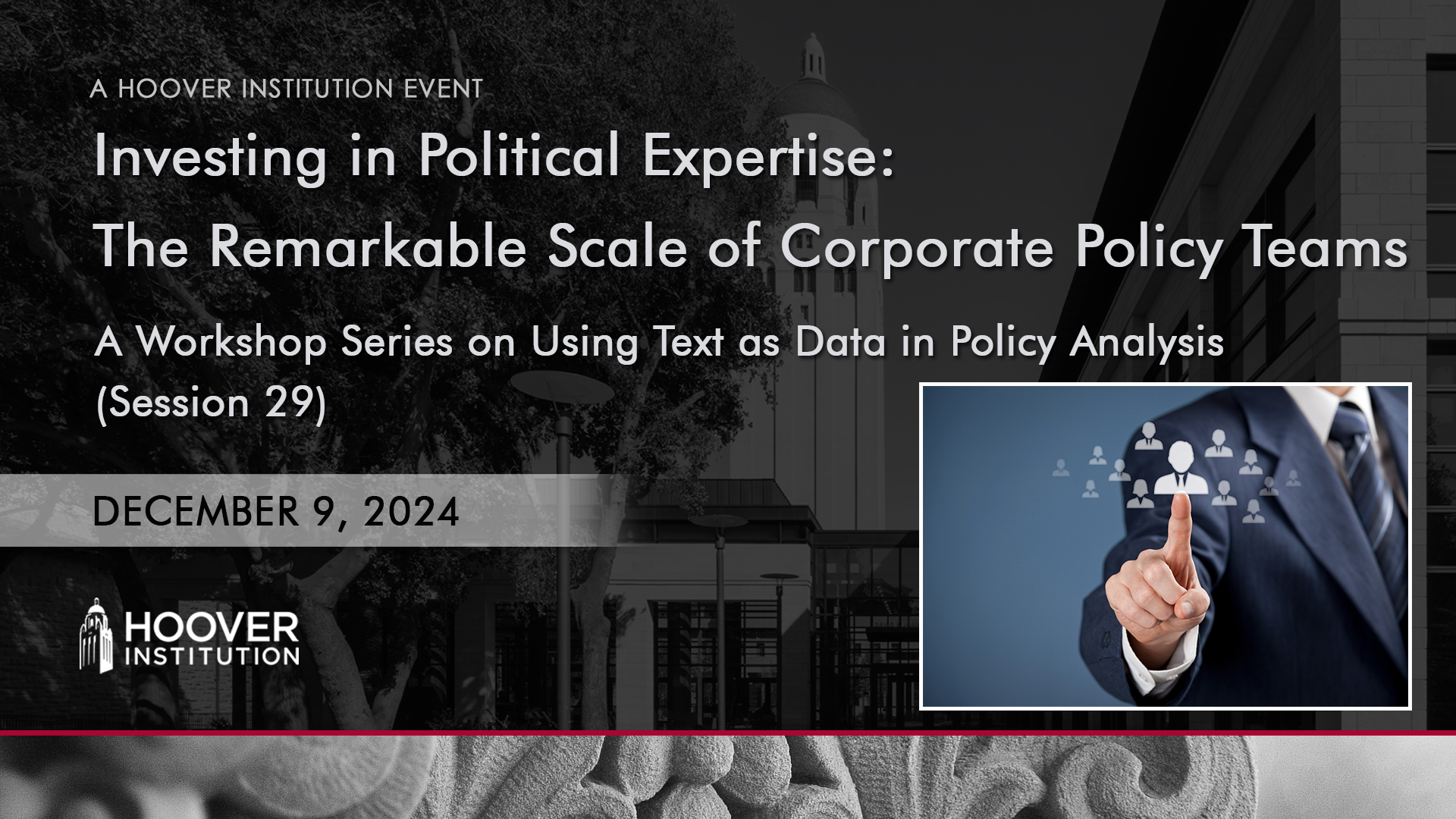
ABOUT THE SPEAKERS
Andrew B. Hall is the Davies Family Professor of Political Economy at the Stanford Graduate School of Business and a Senior Fellow at the Hoover Institution. Hall’s research team uses large-scale quantitative data and cutting-edge methods from econometrics, statistics, and computer science to study American democracy and the design of democratic systems of governance in the online and offline worlds. Hall serves as an advisor to Meta Platforms, Inc and the a16z crypto research group.
Steven J. Davis is the Thomas W. and Susan B. Ford Senior Fellow at the Hoover Institution and Senior Fellow at the Stanford Institute for Economic Policy Research. He studies business dynamics, labor markets, and public policy. He advises the U.S. Congressional Budget Office and the Federal Reserve Bank of Atlanta, co-organizes the Asian Monetary Policy Forum and is co-creator of the Economic Policy Uncertainty Indices, the Survey of Business Uncertainty, and the Survey of Working Arrangements and Attitudes. Davis hosts “Economics, Applied,” a podcast series sponsored by the Hoover Institution.
Erin Baggott Carter is a Hoover Fellow at the Hoover Institution at Stanford University. She is also an assistant professor in the Department of Political Science and International Relations at the University of Southern California, a faculty affiliate at the Center on Democracy, Development and the Rule of Law (CDDRL) at Stanford University’s Freeman Spogli Institute, and a nonresident scholar at the 21st Century China Center at UC San Diego. She has previously held fellowships at the CDDRL and Stanford’s Center for International Security and Cooperation. She received a PhD in political science from Harvard University.