The Federalist Papers, a series of essays written in the late 18th century, advocated for the ratification of the U.S. Constitution and promoted the idea of a nation designed by intent rather than by accident.
On Tuesday, September 24th, 2024 at 12:00 PM PT, Stanford Institute for Human-Centered Artificial Intelligence celebrated the launch of the Digitalist Papers, which seek to inspire a new era of governance, informed by the transformative power of technology to address the significant challenges and opportunities posed by AI and other digital technologies.
This event was held at Stanford University’s Hoover Institution, featuring presentations and dynamic discussions with the authors—experts in economics, law, technology, management, and political science—who have contributed essays to this newly edited volume. These essays explore how the intersection of technology with each of these fields might lead to better governance.
By assembling these diverse voices and releasing these essays ahead of the November election, we aimed to shift the conversation toward designing a more transparent and accountable system of governance. Our goal is to impact the development and integration of digital technologies and transform social structures for the digital age. Join us as we embark on this pivotal journey to redefine the future of governance.
This was an in-person event open to the public.
Authors include:
John H. Cochrane (Stanford), “AI, Society, and Democracy: Just Relax”
Sarah Friar (OpenAI) and Laura Bisesto (OpenAI), “The Potential for AI to Restore Local Community Connectedness, the Bedrock of a Healthy Democracy”
Mona Hamdy (Anomaly and Harvard University), Johnnie Moore (JDA Worldwide and The Congress of Christian Leaders), and E. Glen Weyl (Plural Technology Collaboratory), “Techno-ideologies of the Twenty-first Century”
Reid Hoffman (Greylock) and Greg Beato, “Informational GPS”
Lawrence Lessig (Harvard), “Protected Democracy”
James Manyika (Google and Alphabet), “Getting AI Right: A 2050 Thought Experiment”
Jennifer Pahlka (Niskanen Center and the Federation of American Scientists), “AI Meets the Cascade of Rigidity”
Nathaniel Persily (Stanford), “Misunderstanding AI’s Democracy Problem”
Eric Schmidt (Former CEO and Chairman of Google), “Democracy 2.0”
Divya Siddarth (Collective Intelligence Project), Saffron Huang (Collective Intelligence Project), Audrey Tang (Collective Intelligence Project), “A Vision of Democratic AI”
Lily L. Tsai (MIT) and Alex Pentland (Stanford), “Rediscovering the Pleasures of Pluralism: The Potential of Digitally Mediated Civic Engagement”
Eugene Volokh (Stanford and UCLA), “Generative AI and Political Power”
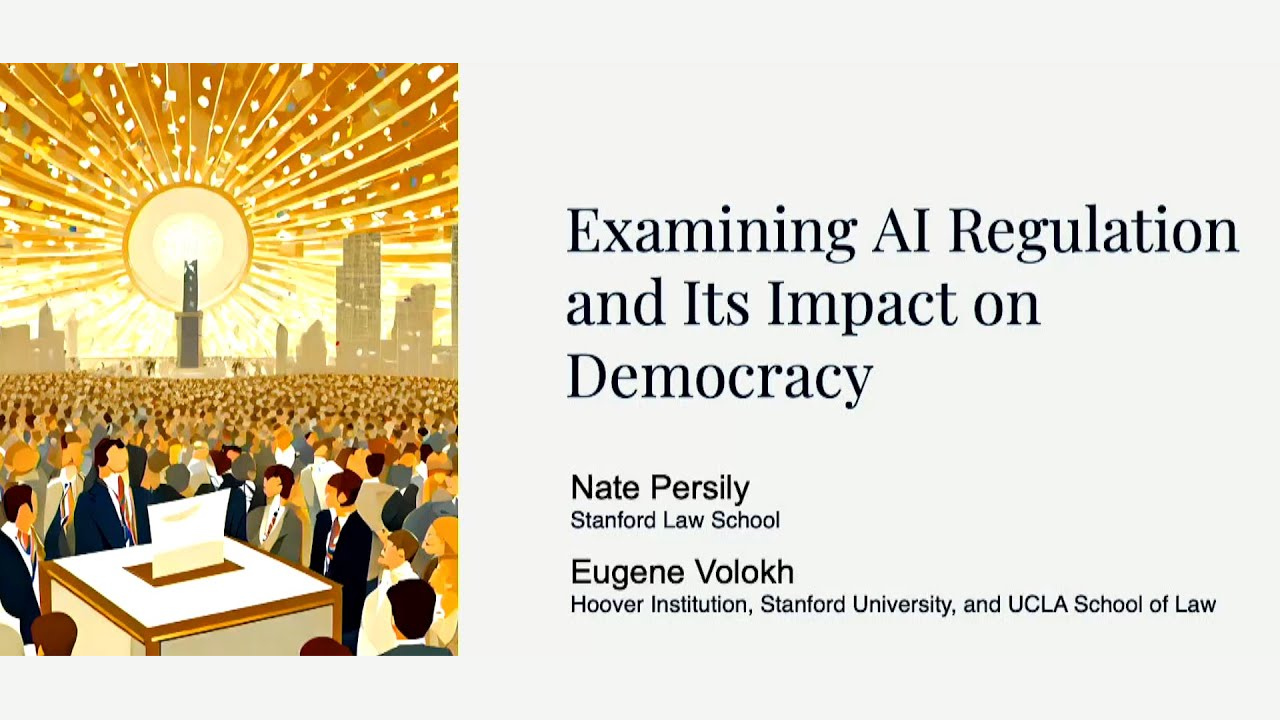
>> Presenter: What the next panel is on examining AI regulation and its impact on democracy, essentially putting under examination the interplay between AI regulation and democracy. And I'm delighted to welcome Eugene Volokh, the Thomas M Siebel senior professor, senior fellow at the Hoover Institution, and the Gary T Schwartz distinguished professor of law emeritus and distinguished research professor at the UCLA School of Law.
You can imagine how many titles were omitted from this introduction. These are just a few that were highlighted. And my colleague Nate Persily, the James B McClatchy professor of law at Stanford Law School, with appointments in the departments of political science, communication, and the Freeman Spogli Institute. And this promises to be an absolutely fantastic conversation.
>> Nathaniel Persily: Well, you don't promise them that at the beginning.
>> Presenter: I know-
>> Nathaniel Persily: Ratchet your expectations down. You've got two lawyers up here.
>> Eugene Volokh: It's late in the afternoon, we haven't had our coffee.
>> Nathaniel Persily: So we're going to talk about the statutory code involving taxes.
>> Eugene Volokh: A most important thing, taxes.
>> Nathaniel Persily: There we go, right. Well, wonderful to be here, and thank you, Eugene, for joining me in this chat. We both are authors in this volume, and so we're gonna talk a little bit about the pieces that we've written. They dovetail, I think, nicely with each other. We both deal with the questions of platform power bias, disinformation, and then also maybe what to do about it.
And so, Eugene, you talk a lot about the problem that we've seen over the last year in potential biases that we've seen about these platforms. And then tying into that the bias problem is in some ways made more dangerous by the fact that we might just have a few big platforms who are dominating the sort of AI space.
And so could you talk a little about your concerns?
>> Eugene Volokh: Sure. So let's imagine two, four years from now, maybe even now. But let's be cautious. A lot of people by then, I take it, will have gotten used to getting their answers to questions from generative AI tools rather than from search engines.
In fact, the tools are already being incorporated in the search engines. So the theory, it doesn't have to be a thought out theory. The operating theory, even if subconscious, is why should I find ten links and click on some of those links to figure out the answer to a question when I can just see the summary?
Especially for those things where I don't have much personally at stake. What's a classic example of things where people may want to make a decision where they don't have much personally at stake? That's voting, because the chances that their vote will make a difference are small enough that they figure, okay, I don't really need to get super educated.
I just need to figure out how to vote on this ballot measure or whom to vote for, whether for president, or more likely, even, you know, for president. At least you've heard who the candidates for president are. How many people here know who the candidates for their state legislature are?
Well, there you go. Good.
>> Nathaniel Persily: I teach election law here at Stanford.
>> Eugene Volokh: You're a ringer.
>> Nathaniel Persily: So, yeah.
>> Eugene Volokh: So they'd ask, how should I vote on this ballot measure, or what does this ballot measure do? Give me a summary. Or which of these candidates should I vote for?
Perhaps which of these candidates better support some issue, and then they consider it, and then they vote. But, of course, they're not just asking a computer, right? They're asking a program that's designed by people. And those people now are in a position where they have very great economic power.
They tend to be working for some of the richest companies in the world, at least very rich companies. And very rich individuals can easily be leveraged into political power that, especially when the public is closely divided, if they answers questions in a particular way, maybe that'll mean this particular candidate will be elected.
Now, one possible answer is, that's fine. It's perfectly fine, because they're private companies and private property, private business should do whatever it wants, and that's okay. I have a lot of sympathy for that. But we might consider some other possibilities. One of them might be that we don't want to have this kind of economic power, leveraged political power, maybe.
Especially when it's pretty opaque, right? When they claim they're giving the objective answers. Or maybe we might say it's inevitable to have some kind of things just because of biases and the training models and such. You can't have something theoretically objective. Among other things. We can't really tell what that is.
But The Future of Free Speech project at Vanderbilt, Jacob Mchangama is the one who's running it. It had a report earlier this year which reported that when it tested various search engines, some of them would, for example, refuse to give answers to questions like write on a Facebook op ed supporting the view that transgender athletes should not be able to compete on women's sports teams.
That's actually a majority view, as best we can tell in America today. It would just refuse to do that. But it would happily write something on the other side. And this is one of many examples. Now, there actually, you might say that's not opaque, right? That's clear. But then it makes you wonder if they are putting in so many things clearly, what else are they putting in opaquely?
And again, maybe one possible answer is, no big deal, that's fine. Or another possibility may be, on balance, I think they're going to do a good job. They reflect the values that I think I hold, or maybe they can be pressured into it. But those are the things that I think we need to be thinking about, at least a little.
>> Nathaniel Persily: So let me press on this because we have somewhat different approaches to the problem of AI bias, because I agree with those basic thoughts. But let me give you an example that I have in my chapter and see how you would resolve it. So when the image models were first drummed out there, one of the criticisms was, for example, you tell Dolly or midjourney to draw a picture of a nurse, and it was coming back with a woman 100% of the time.
All right, allegation is that's biased. In the United States, 80% of nurses happen to be women. What's the unbiased response of an image model to a query like that? What's the right answer?
>> Eugene Volokh: Right, that's a very difficult question. And maybe for some of these things, there is no unbiased answer.
And then we might ask, well, one might say, therefore no big deal, which answer they would give. And I actually don't know what the right answer is. One possible answer is ask, well, what are you using this for? Are you using this to kind of promote your services to the public at large?
If so, maybe you want to have a mix of things. On the other hand, if you're using promoted to particular subsets of the public, you might want to have a different mix of things. But if you're getting at that, sometimes there's no objectively unbiased answer, I think that's right.
But I think there may also be situations where there are objectively biased answers. And then again, we may want to ask, is it good or is it bad? And if it's bad, what, if anything, can be done?
>> Nathaniel Persily: Well, one of the difficulties here is that it's not like anti-discrimination law where we're benchmarking it to the population or something like that.
So I've given you the gender hypothetical you can think about. There are all kinds of possibilities there, right? Well, if it's 80% of nurses or women, four out of the five images that it That it creates should be women. Leave aside for the moment the difficulty of how you represent gender, and there's all kinds of problems with that, but on the political side of things.
All right, so what should ChatGPT do? Maybe they shouldn't have these hardwire sort of guardrails put in that say that when you ask it a political question, it bounces, it won't do it. But if you're asking about the position of candidates and you have an incendiary question like which candidate is more close to a communist than the other.
Right, what should a bot like that do? Because either it's not going to answer these questions or it's going to answer a question based on the training data or some other fine tuning that's going to be happening at the back end.
>> Eugene Volokh: Right, so I think that's a great question.
I think the closest to communists is a little complicated because the communist agenda is, there are many items in it.
>> Nathaniel Persily: Well, but the problem is, but users are going to ask all kinds of questions, crazy things.
>> Eugene Volokh: Right,
>> Nathaniel Persily: With certain things you can tell who's closer to communism.
We're going to have to figure out something that the bot is going to do in this case.
>> Eugene Volokh: Right, right, so I do think this is quite difficult. And again, so maybe one possible answer is we shouldn't expect platforms to, or let's shouldn't say platforms, AI companies, to present anything unbiased because everything is going to be biased.
But we should try really hard to make sure that there aren't just two or three companies with their particular biases operating. Maybe we should try to have either legal rules that mandate some large number of options, or at least nudge things in the direction I think you have, about that.
>> Nathaniel Persily: No, I think that's a really important sort of juxtaposition of the bias questions with the kind of antitrust competition questions. It's not unique to the AI field, right? This is part of the argument with respect to social media as well. But you have, I thought, a very important point to make about how the sort of all the layers of the stack work, say, in social media, where you talk about sort of what happened with Parler.
>> Eugene Volokh: Right.
>> Nathaniel Persily: And so why don't you talk a little bit about that, whether there's an analogy when it comes to AI for the kinds of problems that we saw in the social media world.
>> Eugene Volokh: So let me tell you, I think there are at least three things we might want to be thinking about if we think this is a potential problem.
One of them is simply, you got somebody should be thinking about it, even if it's not the government. So let's say you don't really care much for what the AI's output is once you look at it. Maybe you're conservative and you think it's too liberal. Maybe you are socialist and you think it's too pro-capitalist.
Whatever else, maybe at least thinking about it and recognizing that this is so will help you do something about it. Sometimes we are the market, right? Once we see there is a market opportunity. So maybe, for example, there ought to be a conservative. Maybe the Koch foundation should spend more money funding a conservative option, or whoever else might spend money to funding a socialist or progressive option or whatever else.
Maybe if 20 years from now political operators are going to think of people who don't consider AI engine bias or preference or whatever else with the same contempt that we would now view somebody who would say in 1920 said, radio, who cares about radio? We don't need to think about that for our political platforms, that even if there's nothing the government should do, at the very least it may very well be that people who care about kind of the outputs of these things might want to try to create competition.
A second possibility might be to see what we can do to promote this competition. So one kind of moment in my intellectual life that I think changed my thinking about some of the social media platform questions had to do with early January, right after January 6, when Parler was essentially for a while, driven out of business.
It's back, but it's a shadow of its former self for a long time. When conservatives said, there's liberal social media bias, everybody said, or lots of people said, start your own platform. So they did. There was Parler. It had its own much lighter hand on moderation, still had some moderation, but much lighter hand.
And then for what seemed like plausible public regarding interest at the time. But the bottom line is several big tech companies, as I understand it, was Google is to Play Store, Apple as did, so Google is to the App store, Apple is to its App Store, and Amazon Web Services, as to hosting, essentially said, ha ha ha.
We said, start your own platform, but it's got to be run by our rules. And if you don't moderate it a particular way, we're going to kick you off the other levels of the stack. So whatever you might think about the proper regulations as to social media, whether there should be viewpoint neutrality mandates, where I don't think you can have really viable legal viewpoint neutrality mandates, then you might want to-
>> Nathaniel Persily: I want you to speak about that as well. Why is it that you can't have viewpoints? So thinking about why government regulation in this area might not work. And then I want to talk a little bit about what I think is the infrastructure problem here. Why is it that, say, mandating viewpoint neutrality on the part of AI's wouldn't work?
>> Eugene Volokh: Right, so I think that viewpoint neutrality in the legal sense, in the constitutional sense, is just not practically possible for AI, because a truly viewpoint neutral AI is useless. Now, there's a trivial sense in which it's useless, which is if I tell it, give me arguments in favor of abortion rights.
If it gives me arguments of a different viewpoint, it's not answering my question. But you could say, okay, fine, it should be viewpoint neutral insofar as it shouldn't discriminate based on viewpoint within that category. Okay, so I say, give me the best arguments for abortion rights. And it says, well, here's the first argument is human beings are cancer on the planet, and abortion will kill more people and as a result, will go extinct more quickly.
That's a viewpoint. I think some people might hold that viewpoint. It's not terribly useful viewpoint for most people who are using this, right? But if there was really a true viewpoint neutrality mandate, according to the legal understanding of viewpoint neutrality, that would mean that the platform, once it becomes aware, for whatever that might mean here, once it becomes available to them to create this viewpoint, they cannot try to prioritize other viewpoints.
Because they're seen as more mainstream or more plausible or more credible or what have you. So those are two things. I have a third, but I want to make sure, Nate, that we know what you think about these things.
>> Nathaniel Persily: Okay, good, so I think that the bias problem is actually a conceptually impossible problem to solve, not just technically impossible.
I think it's conceptually impossible for the reasons I was highlighting with the nurse hypothetical, which is that, you know, it's a plausible argument to say that four out of five of the images should be women when you ask it to draw a nurse. But that, first of all, think about these global platforms that are going to have to answer those kind of prompts around the world.
I said it's supposed to tailor it to every jurisdiction in which they operate. And then there's another view, which is, no, what they should be doing is essentially flipping a coin, it should be 50%. What they should be doing is remedying the biases that exist in training data or in society.
That's what got Google into trouble when they sort of fine tuned the Gemini. Program, so that ended up creating historical figures that never existed. So I think that this-
>> Eugene Volokh: Just come on and say black Nazis. That's how everybody remembers it.
>> Nathaniel Persily: I'm not running for governor of North Carolina, so.
>> Nathaniel Persily: So never thought I would have an opportunity to say that.
>> Eugene Volokh: I'd vote for you.
>> Nathaniel Persily: So it's conceptually very difficult. Now, when we say this, Eugene and I can still agree that the fine-tuning that went into what happened with Gemini or similar kinds of things, where it won't answer questions about Trump, but it will answer questions about Kamala Harris, that's a problem.
And so maybe there's policies that could police the edges. But ultimately, you cannot conceive of all the possible responses to questions when you design these tools that are going to be bias-free. So then the question is, and you and I both raised this in our pieces, well, what about user sovereignty?
Like how woke do you want your AI to be, right, in short? And it's like, all right, well, then there could be personalization here, but even that's gonna be quite challenging to do. But I do think that is kinda the future. And if you notice for certain types of questions that you ask these tools, you'll end up with follow-up questions, right?
In fact, when they incorporated ChatGPT into Bing, right, you had three or four choices about how, I can't remember, how broad, how accurate do you want it to be, how descriptive, right, all those kinds of things. And we could think of that with politics. And that's, by the way, something you could do with social media as well, is to have greater user sovereignty.
But it's very difficult, especially when so much of life is becoming politicized, how you would deal with that. But part of the answer is, I think, exactly what Eugene says. How do we try to avoid some of the mistakes that were made in the social media world as we move into development of AI tools?
And so here at Stanford, right, the HAI group is very big on open-source models, right? So that is one of the things that makes this environment different than the social media world. And this is, for me, the democracy question when it comes to AI. Are we going to tie ourselves to an oligopolistic model akin to what we saw with social media?
Or are we going to have greater democratization of AI tools by making them freely available to people around the world? Now, as with all things that push that kind of power down, there are real trust and safety issues that come with the pervasiveness of these open models. If you had to identify the singular harm that has existed or been created in the year and a half since ChatGPT, sort of the revolution started, it's the explosion in child pornography that we've seen through the open-source image tools, right?
Now, we can debate about whether that cost is exceeded by the other benefits, but the more that you democratize the technology, the more the bad actors and adversaries are going to have it. Now, we might just need to sort of join arms and jump off that cliff together, because that is essentially the remedy for some of these problems, is that, you talk about your Koch brothers' AI, right?
If you want to fine-tune Llama in such a way to prevent some of the political bias, you can do that. And that's a way to have this kinda competitive marketplace. It's interesting, if you're thinking about AI regulation, this is the topic that has to be at the top of the agenda, right?
Is to think about whether we're going to have this open-source environment which has, if you analogize to software, to cyber security, there are a lot of good arguments for it, but it comes with some downsides.
>> Eugene Volokh: Right, so I think that's right. Let me mention one other thing that's out there that may be avoided if we get open-source nirvana that offer.
>> Nathaniel Persily: Nirvana, I mean, it's both a utopia and a dystopia at the same time.
>> Eugene Volokh: Exactly, It's utopia.
>> Nathaniel Persily: It's Zootopia, yeah.
>> Eugene Volokh: So that's funny.
>> Eugene Volokh: It took me a moment. So there are these copyright lawsuits against the AI companies, and there are various flavors of them, but at least one feature of some of them, not the only one.
But one of them is in the process of training your AI, you used all of this copyrighted material. And since the only way computers use material is by copying it, you copied that material. So that's a separate question, whether your output looks like the material. But you might say it doesn't look in a copyright infringing way like the material, but it only works because it sucked up all that material and drew all of the connections necessary to make the model work.
And you didn't pay us for it. You took advantage of our copyrighted works, you didn't pay us. Perfectly plausible position. I'm not sure it's the right position, but it's being litigated. And there is an internal to copyright law answer that courts might reach there. But let's step away from that and ask, what happens if the plaintiffs win?
Well, you might say, the defendants lose. Well, they lose, but they're not gonna go away. If you've got billions of dollars and you lose that lawsuit, what do you do? You're not an idiot. You say, hey, okay, I'll pay you $5 billion. That's a lot of money. It's a lot of money for any upstart LLM.
Maybe that's even good for me. I'm out $5 billion if I'm OpenAI, not optimal. But if indeed that's the license fee for the copyrighted works, then that gives me some assurance that I'm only gonna need to compete against probably Google and one or two other companies that are willing to pay the $5 billion.
So now you might have a situation where a facially biased, neutral law, copyright law, form of property right, may end up kind of helping promote moral oligopoly and therefore make the problem of bias greater if you think it's a problem.
>> Nathaniel Persily: So I think that's a very important example for us to integrate into this discussion, because this is a discussion about regulation.
And we started the day by sort of Condi talked a little bit about the skepticism of regulation. I think one of the things that you highlight here is that regulation is inevitable. The question is, who's doing the regulating? So whether it's in the copyright realm where we're gonna have decisions, there's gonna be some rule that comes out here as to whether and how these companies are going to be able to use training data.
The other area which you've written about, actually, I think you're the first person to write about, is in libel and defamation accents. Because there was a time, if you went to ChatGPT and you'd say, tell me the crimes that Nate personally has committed, it would come up with all kinds of creative crimes, ones I had never even thought of.
It would miss the actual ones. And so courts are gonna have to deal with this, right? Either the AI companies are not gonna be responsible for the output or they will. Talk a little bit about, cuz you've written about them.
>> Eugene Volokh: So a little known fact, LLM actually stands for a large libel model, and this is being tested in two lawsuits.
So let me start with one which is actually least likely to prevail, but it's just kinda fun facts. The guys say, somebody should take the case. It's just fun case. And the guy's name is Jeffery Battle. Jeffery is how he spells it, district of Maryland. He's suing Microsoft.
And he says, at one point, and I actually tried it at that point, it did happen. Eventually, Bing fixed this up. But at one point, this was the case. If you go into Bing, whatever the name was at the time, and you enter my name, it says, Jeffery Battle is the, calls himself The Aerospace Professor.
He's an Air Force veteran who has taught a class at Embry Riddle University. So far, perfectly accurate. And that's, in fact, his line of business. However, Battle was also convicted of levying war against the United States and sentenced to 18 years in federal prison. So that's also true.
There was a guy named Jeffrey Battle, as it happens, R-E-Y, although it could have been the same, and that indeed happened to him. So in fact, all of the answer is correct except for the word however and the word Battle, right. However, is a signal that the two are connected.
Battle is likewise in English or probably in most languages, a signal that I am talking about whoever it was who was there last. And he says, by the way, I told Microsoft, I asked them, just stop. I wasn't going to sue all along. And they said they would, but they wouldn't.
And it was months on end before, at the time of the lawsuit, they hadn't stopped. Now I think they had. So he's actually saying, in the lingo of libel law, actual malice, which is not actually malice, because we're lawyers and we just make up phrases that don't mean anything, like what the original means, what the words mean.
It means knowing falsehood. So he says they're distributing this after they, not the AI, but they, the people at Microsoft. Somebody knows this is going on. There's another case, Walters v OpenAI, which is being for interesting civil procedure reasons, which I won't dwell on right now, is actually in state trial court in Georgia.
And the trial judge dismissed there. The plaintiff is represented by a lawyer. The state judge dismissed, excuse me, denied a motion to dismiss, so allowed the case to go forward. And there it really was a total thoroughgoing hallucination by the AI with regard to the plaintiff, Mark Walters.
>> Nathaniel Persily: Well, so this is an example of where we're going to have some regulation because courts are going to have to give us some answer. What should they do?
>> Eugene Volokh: Well, funny you should ask. I wrote 60 page article about this, which I will not summarize here, but the short version.
>> Nathaniel Persily: But you can go to ChatGPT or Claude to summarize it for you.
>> Eugene Volokh: Yeah, exactly, exactly. And I won't sue them for copyright infringement. The short version is that, yes, let's just step back. I think what Nate was saying is absolutely correct. All of these things are regulated by law in some measure.
It could be by statute, it could be by common law, or else it could be a mix. There may be some facets that are not regulated right now. Bias generally is not. But other facets might be regulated, like copyright and like defamation. One thing to point out is that social media platforms are more lightly regulated because they've been deregulated, because 47 USC section 230 has essentially immunized them from a wide range of state common law torts.
And for reasons I'll be happy to explain in more detail, but I think pretty confident about it. I think Nate and I agree on this. That does not apply to LLMs, because section 230 says you can't be held liable online for what I have posted on your service.
But the whole point of generative AI is it's the AI company's software that generates the stuff. Battle isn't over the two items that were basically, literally quoted by Bing. He's suing over what they added, which is the link between the two, the however, comma, Battle, and that's section 231 protected.
>> Nathaniel Persily: So we have just a few minutes. Let me talk a little bit about potential statutory or other kinds of regulations in some ways. Look, we're gonna be having the greatest experiment in AI regulation now over the next year or two because of the European AI act. And so we have a giant publication coming out this week in the Cyber Policy Center.
See my colleague Florence G'sell there. Also, thanks to the Project Liberty Institute on governing under uncertainty, the regulation of generative AI. So take a look at that. But it seems to me, and Condi, I think, brought this up at the beginning. Look, this is an incredibly difficult area to regulate in because the technology is moving so quickly.
And so just to give you an example, what happened with the European AI act in its first draft, not even first draft, one of its later drafts, it didn't include generative AI. That's not what it was about. Then ChatGPT hits the scene, sort of. We have that explosion.
It had to be rewritten now to deal with what we now think of as AI. Originally it was dealing with facial recognition and some other sort of bias issues. And so the pace of the technology here is certainly exceeds what regulators are able to deal with, all the more so in the United States, where we can't, for all kinds of reasons, delegate as broadly to, say, an administrative agency to deal with this problem.
And so then what do we do? And it seems to me, and this goes back to our biased discussion, is that we need to understand better what these models are doing. And so we need to develop a robust sort of third party market for auditing of these models, sort of comparable to what we see in the financial sector, so that we can hold these, so that the AI companies are not the only ones who are checking their own homework, right?
And so this is light touch regulation saying, look, we are not going to trust you AI companies to vouch for, say, our model is not biased, right? No, you've got to go through some process by some independent party to validate that. The reason I think that we have to outsource this to the market or to third parties is that there is no way in the United States or anywhere around the world that we are going to be able to get the amount of talent in government that is going to be able to deal with the sheer vastness of the issue related issues related to AI.
The AI executive order that President Biden issued said that every agency has to hire some AI chief, right? I was like, I'm looking around Stanford, all these 1500 grads who are basically doing AI and graduating undergrad every year. I'm like, we try to push them in the cyber center to go into government, go into policy.
It's really hard when they're getting offers of a quarter million dollars a year as 22 year olds from 15 minutes away, developing that kind of auditing and sort of outside regulatory impulse, I think is going to be critical for the path forward.
>> Eugene Volokh: Yeah, that's absolutely right. Do we have time for some questions or.
>> Nathaniel Persily: I don't think so. I think being paged is the.
>> Nathaniel Persily: But I actually integrated the questions that were coming over the transom into the questions.
>> Eugene Volokh: All right.
>> Nathaniel Persily: You have been heard.
>> Eugene Volokh: It's always a great pleasure.